A Comparative Analysis of ENISA and NIST AI Risk Management Frameworks
- THEMIS 5.0
- Aug 16, 2024
- 3 min read
As a project focused on helping people to assess the trustworthiness of AI decisions, we understand that managing associated risks has become a paramount concern for many organisations working with, or thinking of working with AI. To address this challenge, various frameworks have been developed to guide stakeholders in identifying, assessing, and mitigating AI-related risks. Two prominent frameworks in this domain are those provided by the European Union Agency for Cybersecurity (ENISA) and the National Institute of Standards and Technology (NIST) in the United States. In this post, we will undertake a brief analysis of these frameworks to understand their similarities, differences, and applicability in real-world scenarios.
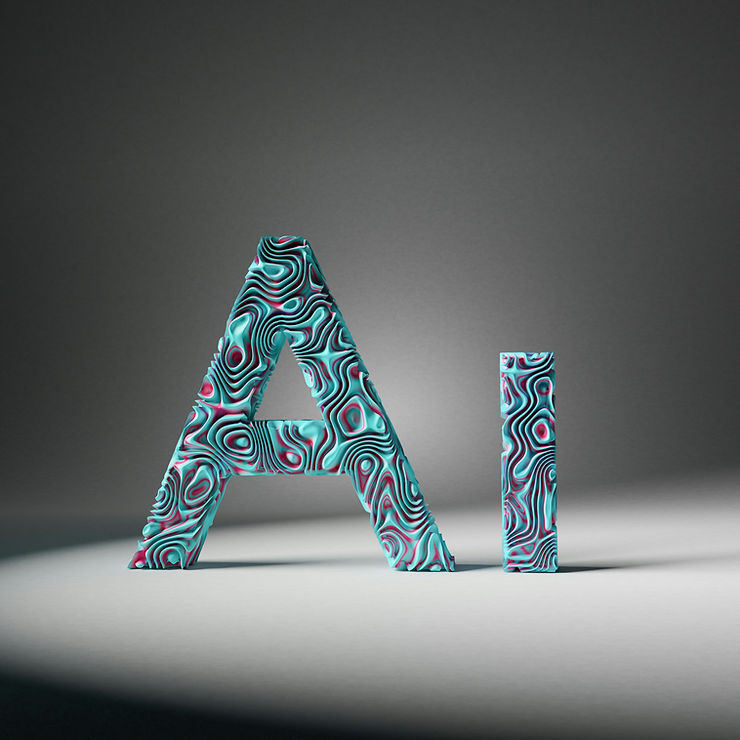
Overview of ENISA and NIST AI Risk Management Frameworks
ENISA AI Risk Management Framework: The European Union Agency for Cybersecurity (ENISA) has developed a comprehensive AI risk management framework that provides guidance to organisations operating within the EU. This framework emphasises the importance of understanding AI systems' lifecycle, from design and development to deployment and operation. ENISA's approach focuses on identifying potential risks at each stage of the AI lifecycle and implementing appropriate measures to mitigate these risks effectively. Key components of the ENISA framework include risk assessment methodologies, regulatory compliance, and ethical considerations.
NIST AI Risk Management Framework: The National Institute of Standards and Technology (NIST) in the United States has also developed a robust AI risk management framework aimed at assisting organisations in managing the risks associated with AI technologies. NIST's framework is based on established risk management principles and consists of several components, including risk identification, risk assessment, risk mitigation, and risk monitoring. Additionally, NIST provides guidelines for integrating risk management processes into organizations' existing frameworks and practices, promoting a holistic approach to AI risk management.
Comparative Analysis:
Scope and Coverage: ENISA and NIST frameworks share a common goal of helping organisations manage AI-related risks effectively. However, they differ slightly in their scope and coverage. ENISA's framework places a strong emphasis on regulatory compliance and ethical considerations, reflecting the EU's regulatory landscape. On the other hand, NIST's framework focuses more on technical aspects of risk management, providing detailed guidelines for risk assessment and mitigation strategies.
Risk Assessment Methodologies: Both frameworks advocate for the adoption of risk assessment methodologies to identify and prioritise AI-related risks. ENISA's framework emphasises the importance of contextual risk assessment, taking into account factors such as the AI system's intended use, potential impact, and stakeholders involved. In contrast, NIST's framework provides more prescriptive guidance on risk assessment techniques, such as threat modelling and vulnerability analysis, enabling organisations to conduct thorough risk assessments.
Implementation Guidance: NIST's framework offers comprehensive implementation guidance, including practical recommendations for integrating risk management processes into organisations' existing structures. This approach facilitates the adoption of risk management best practices and ensures alignment with established standards and frameworks. ENISA's framework also provides implementation guidance but places greater emphasis on regulatory compliance and ethical considerations, reflecting the EU's regulatory priorities.
We believe that both the ENISA and NIST AI risk management frameworks offer valuable guidance to organisations seeking to manage AI-related risks effectively. While ENISA's framework prioritises regulatory compliance and ethical considerations, NIST's framework focuses more on technical aspects of risk management. Ultimately, the choice between these frameworks depends on organisations' specific needs, regulatory requirements, and operational context. By leveraging the insights provided by these frameworks, organisations can develop robust risk management strategies to navigate the complex landscape of AI technologies securely.
Comments