Unleashing the Power of Machine Learning
- Bella Callaway
- Mar 12, 2024
- 3 min read
In today’s rapidly evolving technological landscape, the term ‘machine learning’ has become part of the everyday vocabulary for many across the globe. From optimising business operations to revolutionising healthcare, the applications of machine learning are seemingly limitless. But what exactly is machine learning, and why is it garnering so much attention?
At its core, machine learning is a subset of artificial intelligence that enables systems to learn from data and make decisions or predications without being explicitly programmed. Instead of relying on rigid instructions, these systems utilise algorithms to analyse vast amounts of data, recognise patterns, and make informed decisions. The beauty of machine learning lies in its ability to continuously improve and adapt as it encounters new data, thereby enhancing its predictive accuracy over time.
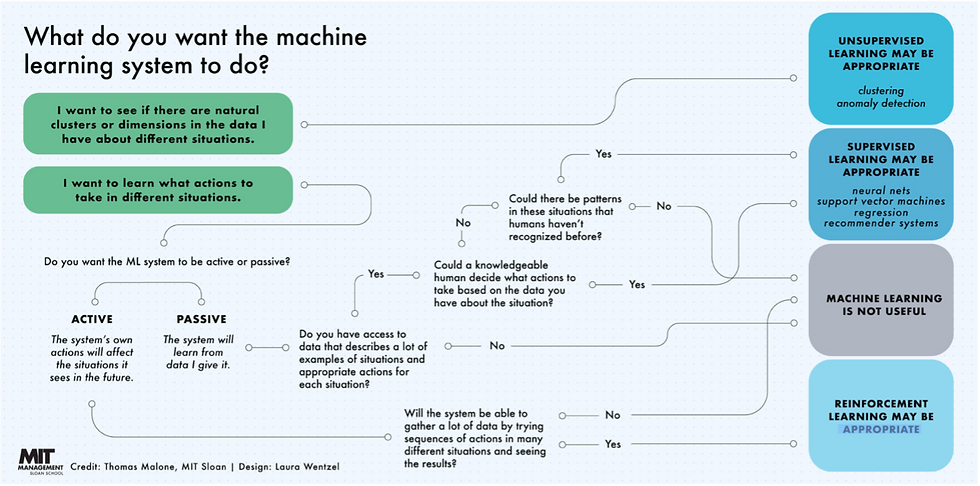
Flowchart demonstrating how humans can utilise ML via MIT Sloan
There are several key components that form the basis of machine learning:
1) Data: At the heart of every machine learning tool is a significant quantity of data. Data serves as the raw material from which patterns can be identified and then analysed. Whether its images, text, numerical values or sensor readings, the quality and quantity of data playa. Crucial role in determining the effectiveness of a machine learning model.
2) Algorithms: Algorithms are responsible for learning patterns that are found in data and making predictions or decisions based on this analysis. There are various types of algorithms, each designed to suit a different type of task.
3) Training: Like human workers, a machine learning model undergoes training to develop its abilities. During the training process, the model is fed labelled examples from the data, allowing it to learn the underlying patterns and relationships. Through iterative adjustments to its internal parameters, the model gradually improves its performance until it achieves the desired level of accuracy.
4) Evaluation: Once trained, the model is put to the test, evaluating its performance. This typically involves feeding it new, unseen data and measuring how well it generalises to make predictions or classifications. By comparing its predictions to already known outcomes, users can assess the model’s accuracy and identify areas for improvement.
5) Deployment: finally, the trained model is deployed into production, where it can be used to make real-time predictions or decisions. The machine learning models hones its craft and has the ability to adapt and evolve over time. As new data becomes available and circumstances change, machine learning models can be re-trained to stay up to date and maintain peak performance. This iterative cycle of learning, evaluation, and refinement lies at the heart of continuous improvement that drives the success of machine learning applications.

THEMIS 5.0: Experimental-driven approach to Trustworthiness Optimisation
THEMIS 5.0 will leverage the intelligence of machine learning by combining it with high level knowledge specifications to create a socio-technical environment in which an AI system operates to allow the user to create a model that aids them in understanding decisions they are making. This simulated model will be transparent to the user and trusted by the user as they understand the environment, they have themselves modelled. The final THEMIS 5.0 ecosystem seeks to involve users in interactive dialogues powered by AI and assist them in determining the level of trustworthiness associated with a specific AI judgement. Essentially, a conversational agent powered by artificial intelligence (AI) will aid in maintaining transparency by offering comprehensible explanations of the decision-making process under evaluation. Additionally, the chatbot will collect data on user values and business objectives to guarantee that the outcomes are pertinent to the needs of the user. Because of this ongoing communication, cycles of trustworthiness improvement are facilitated, enabling consumers to evaluate products in a human-centred manner and developers and suppliers to make necessary corrections.
The combination of a knowledge-based approach in conjunction with machine learning technology ensures that outcomes are more trustworthy as they are approached with a human centred focus rather than a purely machine lens. At its crux, machine learning is a blend of mathematics, statistics and computer science that extracts insights from data to make intelligent decisions. By demystifying the inner workings of machine learning, we gain a deeper appreciation for its capabilities and a clearer understanding of how it can be harnessed to tackle some of the most pressing challenges facing society today.
Comments